Theme: Research
PhD defence of Chengdan Xue
Chengdan Xue defends his PhD in Computational Mechanics and Materials on March 15th, 22
Modeling of grain structure and hot cracking for arc welding processes
Chengdan Xue conducted his PhD work in 2MS team, under the supervision of Charles-André Gandin, Michel Bellet and Gildas Guillemot. Chengdan defends his PhD in Computational Mechanics and Materials on March 15th, 2022 in front of the following jury:
Abstract:
Keywords: welding, cracking, solidification, multi-scale modelling, microstructure, CAFE approach
AFPM2022
- Deadline for poster abstract submission: March 15th, 2022
PhD defence of Saoussen Ouhiba
Saoussen Ouhiba defends her PhD in Computational Mechanics and Materials on Feb. 22nd, 22

PhD defence of Junfeng Chen
Convolutional neural networks for steady flow prediction around 2D obstacles
Junfeng Chen conducts his PhD work in the CFL team. He will defend his PhD in Computational Mathematics, High Performance Computing and Data, on January 27th, 22 in front of the following jury:
– Prof. Emmanuelle Abisset-Chavanne, Arts et Métiers ParisTech
– Prof. Anne Johannet, IMT Mines Alès
– Prof. Jean-Luc Harion, IMT Mines Telecom Lille Douai
– Prof. Elie Hachem, CEMEF Mines Paris, PhD Supervisor
– MA Frédéric Heymes, IMT Mines Alès, PhD Co-supervisor
– IR Jonathan Viquerat, CEMEF Mines Paris, PhD Advisor
Abstract :
Over the past few years, neural networks have arisen great interest in the computational fluid dynamics community, especially when used as surrogate models, either for flow reconstruction, turbulence modeling, or for the prediction of aerodynamic coefficients. This thesis considers using convolutional neural networks, a special category of neural networks designed for images, as surrogate models for steady flow prediction around 2D obstacles. The surrogate models are calibrated in the framework of data fitting, with the data set prepared by high-fidelity solvers to Navier-Stokes equations and projected onto cartesian grids. Once calibrated, the models show high accuracy in terms of velocity and pressure prediction, even around obstacles not seen during the calibration. In the next step, a new architecture of convolutional neural networks is proposed for anomaly detection and uncertainty quantification along with the steady flow prediction, making the surrogate model aware whether it is doing interpolation or extrapolation while doing prediction. With these methods, the user of a calibrated neural network can either decide whether to accept a prediction or not, or have a quantified estimation of the prediction error. The third contribution is to use graph convolutional neural networks as surrogate models to predict velocity and pressure on triangular meshes, which have significant advantages in geometry representation compared to cartesian grids. Thanks to the mesh refinement close to the solid interfaces, the graph-based model can give more accurate boundary layer prediction than traditional convolutional neural networks. The last part of this thesis considers integrating physical knowledge into the calibration of a graph convolutional neural network, which is calibrated by minimizing the residual of Navier-Stokes equations on a triangular mesh. The predicted velocity and pressure around a cylinder are of very high quality when compared to the results of high-fidelity numerical solvers. Being not in the framework of data fitting, this approach provides a novel solver to partial differential equations, and deserves more work on its convergence and computational cost.
Keywords: convolutional neural networks, surrogate models, surrogate models, finite element, uncertainty quantification, graph convolution
PhD defence of Karen Alvarado Vargas
Karen Alvarado Vargas defends her PhD in Computational Mechanics and Materials on Jan. 20th, 22
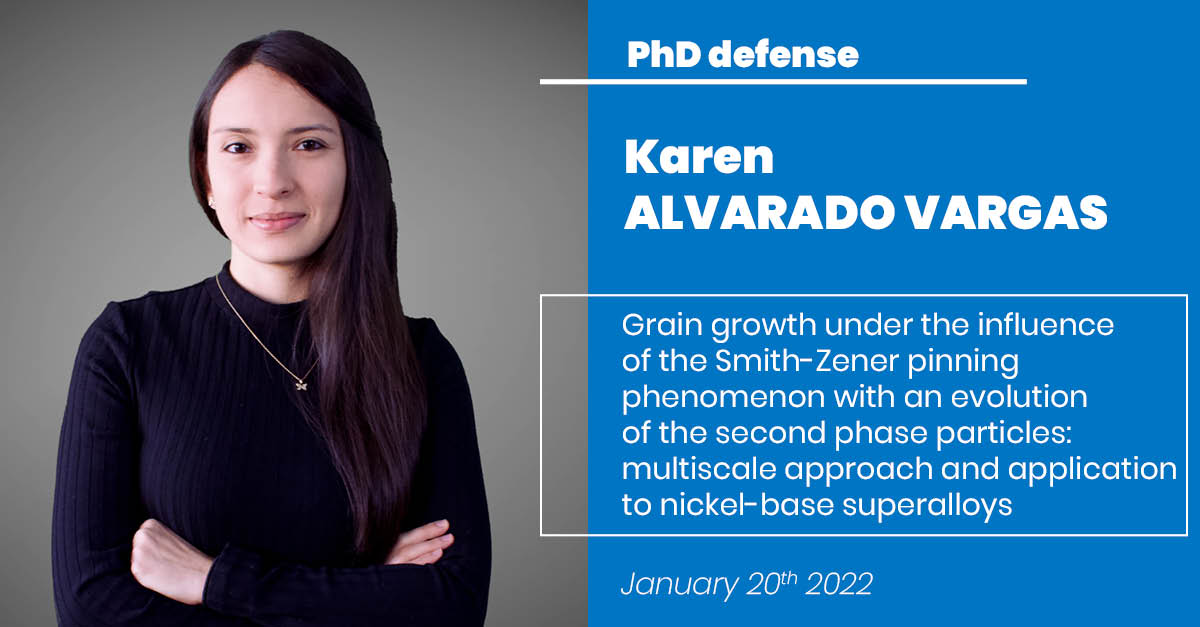
Keywords: Grain growth, Smith-Zener pinning, Level-Set, full-field simulations, 𝛾-𝛾’ superalloys, precipitate dissolution kinetics
PhD defence of Hazem Eldahshan
Hazem Eldahshan defends his PhD in Computational Mechanics and Materials on Jan. 11, 22
3D ductile damage to fracture transition based on phase field and mesh adaptation: application to metal forming processes
Hazem Eldahshan conducted his PhD work in CSM team, under the supervision of Pierre-Olivier Bouchard and Daniel Pino Munoz. Hazem defends his PhD in Computational Mechanics and Materials on Jan. 11th, 2022 in front of the following jury:
Abstract:
Keywords: Ductile fracture, Damage to fracture transition, Phase field model, Adaptative remeshing, Discrete crack propagation, Metal forming applications