Theme: Research
PhD defence de Tiphaine Houdard
Tiphaine Houdard defends her PhD in Computational Mechanics and Materials on July 13th, 22
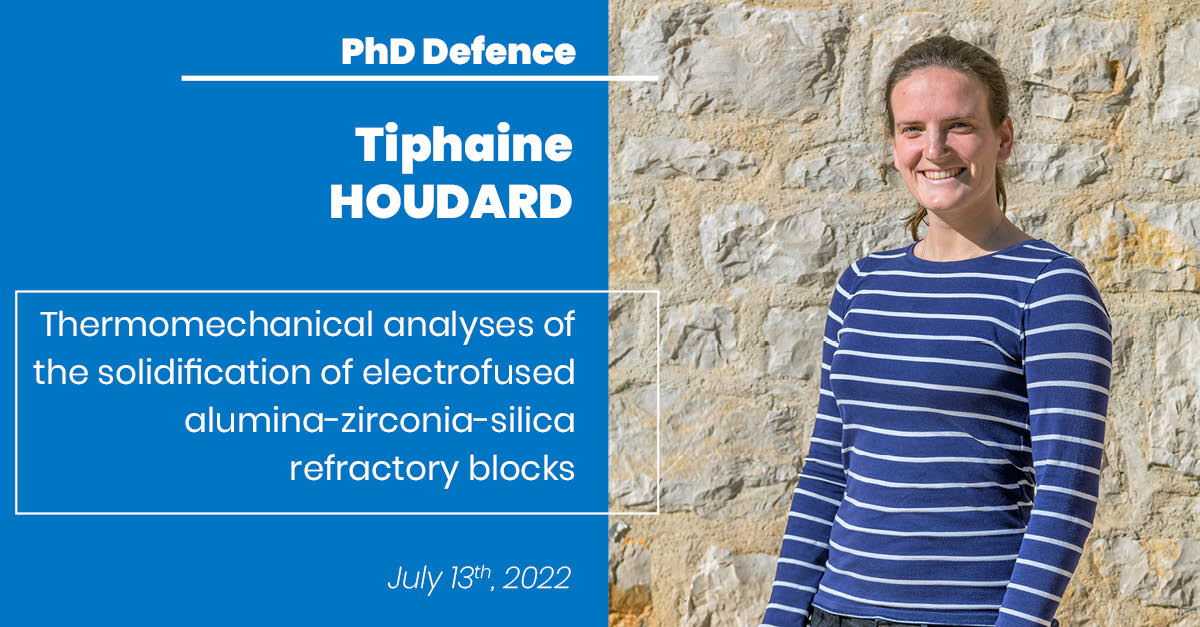
PhD defence of Han Wang
Han Wang defends his PhD in Computational Mechanics and Materials on July 8th, 22.
Study of the solidification of lipsticks and the resultant mechanical behavior. Application of numerical simulation to the forming process.
Han Wangconducted his PhD work in the 2MS and PSF teams under the supervision of Michel Bellet and Séverine A.E. Boyer. He defends his PhD in Computational Mechanics and Materials on July 8th, 2022 in front of the following jury:
Abstract:
Lipsticks are formed by casting process. To understand and improve the industrial casting process, the solidification of model materials of lipsticks are studied by experimental characterization and numerical simulation. Experimentally, the crystallization kinetics of wax components on rapid cooling conditions is characterized and modelled by using the Differential Scanning Calorimetry (DSC). The mechanical constitutive model according to the progress of the solidification process is determined with different rheological testing protocols (Couette rheological tests and indentation tests). The crystallization morphology of different wax components is correlated to different cooling conditions. Numerically, the kinetics model of crystallization and the mechanical behavior law are associated and integrated in finite elements based numerical calculations. Thermomechanical phenomena of the casting process and probable causes of cast defects are analyzed by numerical simulation of the industrial process.
Keywords: lipstick, casting process, crystallization kinetics, mechanical behavior, crystallization morphology, numerical simulation
PhD defence of Ali-Malek Boubaya
Ali-Malek Boubaya will defend his PhD in Computational Mathematics, High Performance Computing and Data on July 5, 22.
Ali-Malek Boubaya conducts his PhD work in the CFL team. He will defend his PhD in Computational Mathematics, High Performance Computing and Data, on June 24th, 22 in front of the following jury:
Keywords: Mathematics, Numerical analysis, Comutational Fluid Dynamics, High Performance Consulting, Thermic
PhD defence of Rémy Gérard
Rémy Gérard will defend his PhD in Computational Mathematics, High Performance Computing and Data on July 4, 22.
Fast, flexible surface to surface thermal radiative transfer for sintering applications
Rémy Gérard onducts his PhD work in the CFL team. He will defend his PhD in Computational Mathematics, High Performance Computing and Data, on July 4th, 22 in front of the following jury:
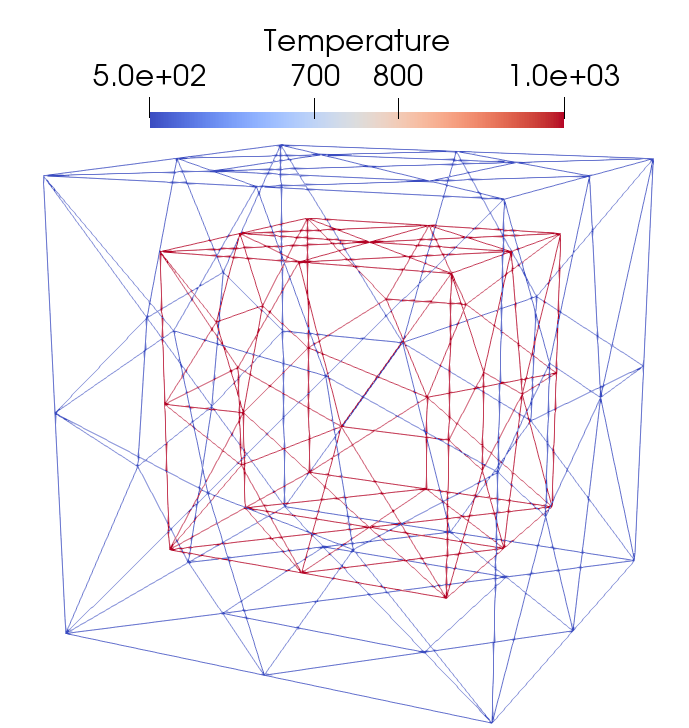
Phd defence of Mohamed Mahmoud
Mohamed Mahmoud defens his PhD in Computational Mechanics and Materials on June 30, 22.
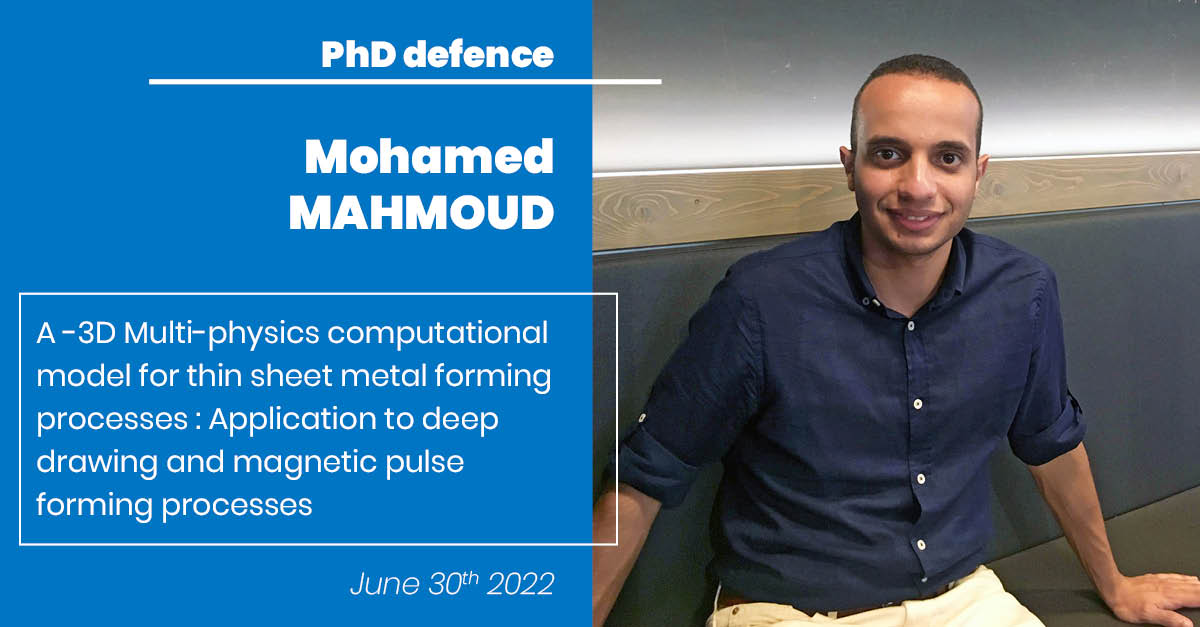
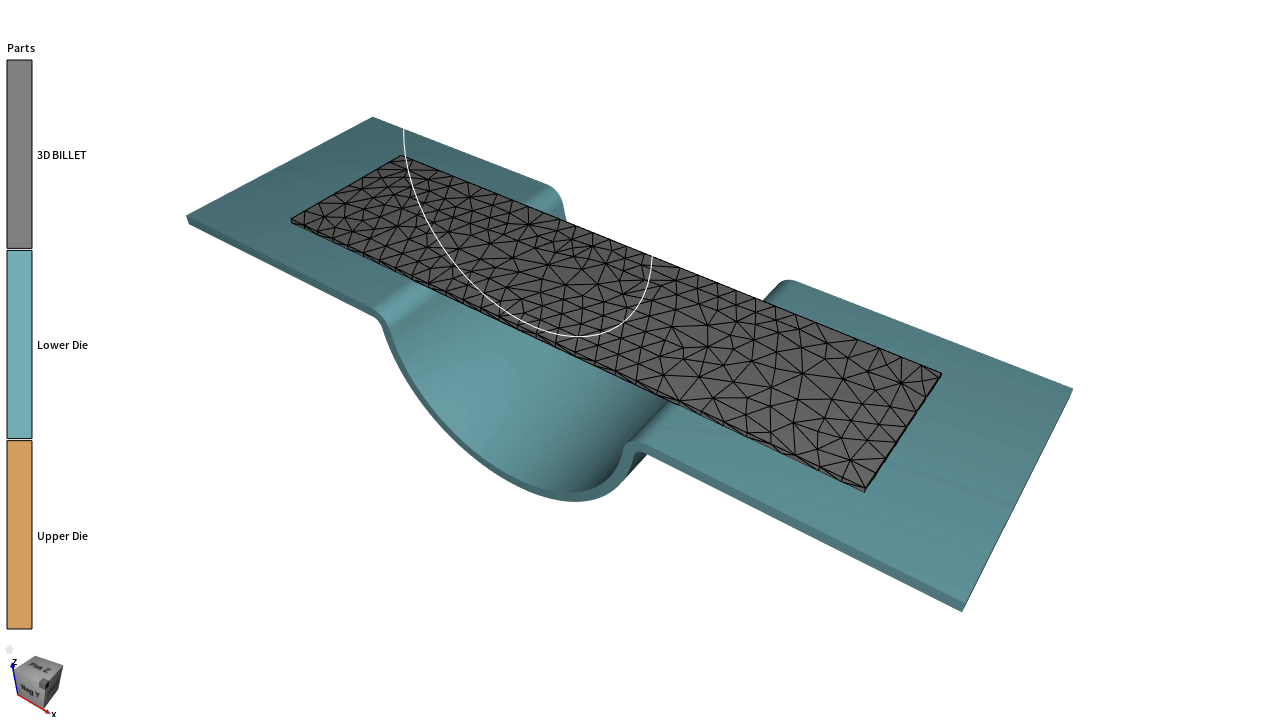
PhD defence of Hassan Ghraieb
Hassan Ghraieb will defend his PhD in Computational Mathematics, High Performance Computing and Data on June 26, 22.
On the coupling of deep reinforcement learning and computational fluid dynamics
Hassan Ghraieb conducts his PhD work in the CFL team. He will defend his PhD in Computational Mathematics, High Performance Computing and Data, on June 24th, 22 in front of the following jury: